Artificial Intelligence Learns Language Translation Without Human Interference
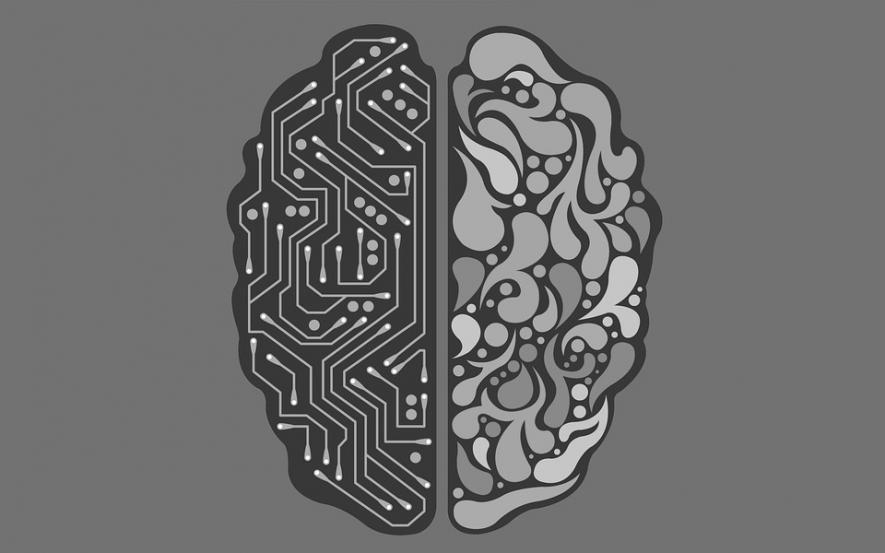
Two teams of computer scientists, one at Facebook, and the other at University of the Basque Country (UPV) in Spain, have made a new breakthrough in translating languages using artificial intelligence. Both groups independently formulated techniques to enable neural networks to translate between two languages without the use of a dictionary or human intervention. Translation has never been done by AI before without training it with translation texts.
Both the techniques first identify patterns in each language individually. For instance, they recognised word pairs which are common across languages, such as table-chair or shoes-socks. The neural networks then linked these co-occurences in both languages and developed bilingual dictionaries without any human feedback on the accuracy of the translation. Later, these dictionaries were used for translating whole sentences.
Neural networks are powerful machine learning algorithms that imitate the structure of the human brain. These algorithms have been trained to perform a variety of complex tasks, from facial recognition to decision making in games such as chess. But these algorithms require training with huge labelled datasets, similar to how our brain learns to perform a new task by acquiring knowledge about it. For the purpose of translation, a neural network will require some parallel texts for it to learn how to perform translation by itself. Parallel texts consist of translated words and sentences placed along with the text in the original language.
But these two new papers have demonstrated how a neural network learnt translation without any parallel texts for training, by the process of unsupervised machine learning.
To translate sentences using the dictionary, the neural networks used two more techniques: back translation and denoising.
Back translation involves a sentence being translated to the required language, and then translated back into the original language. If there are differences between the back-translated sentence and the source sentence, the neural network learns and adjusts itself to make a more accurate translation next time. Denoising follows a similar process, but it also adds some noise to the sentence. The translation is done from one language to the other, then some words are rearranged or removed before it is back-translated into the original language. This essentially takes away the need for human feedback. It also makes the process better than a simple word-for-word translation as it allows the neural network to learn the syntax of both languages, and give better results.
Both papers were published within days of each other and use strikingly similar techniques, and they achieved comparable accuracy. The bilingual evaluation understudy score which is used to measure accuracy of translations was about 15 for both the neural networks. It is lower than humans, who can score more than 50, and also lower than Google Translate, which can score around 40. But Google Translate uses parallel texts and other language data for training.
Both teams have said that the score for their techniques can go much higher when the algorithms are provided with some parallel texts, making it a semi-supervised approach.
Get the latest reports & analysis with people's perspective on Protests, movements & deep analytical videos, discussions of the current affairs in your Telegram app. Subscribe to NewsClick's Telegram channel & get Real-Time updates on stories, as they get published on our website.